November 4, 2024
Empowering systemic racism research at MIT and beyond
Researchers in the MIT Initiative on Combatting Systemic Racism are building an open data repository to advance research on racial inequity in domains like policing, housing, and health care.
Scott Murray | Institute for Data, Systems, and Society.
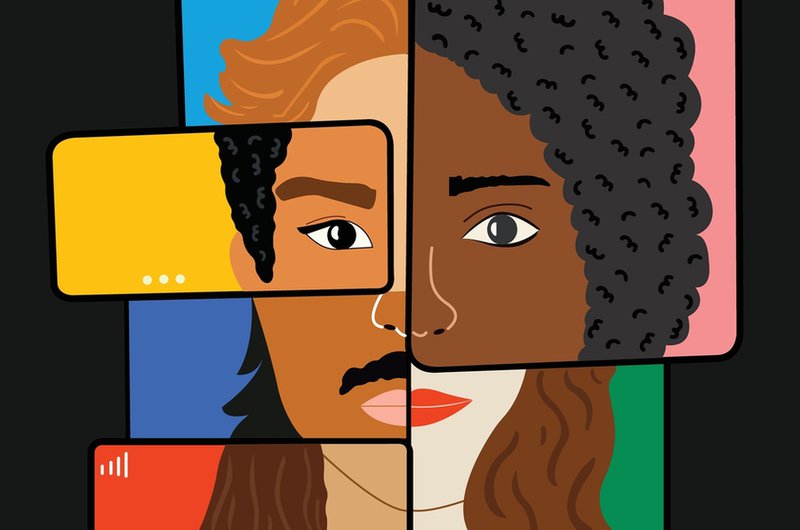
Caption:The new ICSR Data Hub serves as an evolving, public web depository of datasets gathered by MIT researchers examining racial bias in American society and institutions.
At the turn of the 20th century, W.E.B. Du Bois wrote about the conditions and culture of Black people in Philadelphia, documenting also the racist attitudes and beliefs that pervaded the white society around them. He described how unequal outcomes in domains like health could be attributed not only to racist ideas, but to racism embedded in American institutions.
Almost 125 years later, the concept of “systemic racism” is central to the study of race. Centuries of data collection and analysis, like the work of Du Bois, document the mechanisms of racial inequity in law and institutions, and attempt to measure their impact.
“There’s extensive research showing racial discrimination and systemic inequity in essentially all sectors of American society,” explains Fotini Christia, the Ford International Professor of Social Sciences in the Department of Political Science, who directs the MIT Institute for Data, Systems, and Society (IDSS), where she also co-leads the Initiative on Combatting Systemic Racism (ICSR). “Newer research demonstrates how computational technologies, typically trained or reliant on historical data, can further entrench racial bias. But these same tools can also help to identify racially inequitable outcomes, to understand their causes and impacts, and even contribute to proposing solutions.”
In addition to coordinating research on systemic racism across campus, the IDSS initiative has a new project aiming to empower and support this research beyond MIT: the new ICSR Data Hub, which serves as an evolving, public web depository of datasets gathered by ICSR researchers.
Data for justice
“My main project with ICSR involved using Amazon Web Services to build the data hub for other researchers to use in their own criminal justice related projects,” says Ben Lewis SM ’24, a recent alumnus of the MIT Technology and Policy Program (TPP) and current doctoral student at the MIT Sloan School of Management. “We want the data hub to be a centralized place where researchers can access this information via a simple web or Python interface.”
While earning his master’s degree at TPP, Lewis focused his research on race, drug policy, and policing in the United States, exploring drug decriminalization policies’ impact on rates of incarceration and overdose. He worked as a member of the ICSR Policing team, a group of researchers across MIT examining the roles data plays in the design of policing policies and procedures, and how data can highlight or exacerbate racial bias.
“The Policing vertical started with a really challenging fundamental question,” says team lead and electrical engineering and computer science (EECS) Professor Devavrat Shah. “Can we use data to better understand the role that race plays in the different decisions made throughout the criminal justice system?”
So far, the data hub offers 911 dispatch information and police stop data, gathered from 40 of the largest cities in the United States by ICSR researchers. Lewis hopes to see the effort expand to include not only other cities, but other relevant and typically siloed information, like sentencing data.
“We want to stitch the datasets together so that we have a more comprehensive and holistic view of law enforcement systems,” explains Jessy Xinyi Han, a fellow ICSR researcher and graduate student in the IDSS Social and Engineering Systems (SES) doctoral program. Statistical methods like causal inference can help to uncover root causes behind inequalities, says Han — to “untangle a web of possibilities” and better understand the causal effect of race at different stages of the criminal justice process.
“My motivation behind doing this project is personal,” says Lewis, who was drawn to MIT in large part by the opportunity to research systemic racism. As a TPP student, he also founded the Cambridge branch of End Overdose, a nonprofit dedicated to stopping drug overdose deaths. His advocacy led to training hundreds in lifesaving drug interventions, and earned him the 2024 Collier Medal, an MIT distinction for community service honoring Sean Collier, who gave his life serving as an officer with the MIT Police.
“I’ve had family members in incarceration. I’ve seen the impact it has had on my family, and on my community, and realized that over-policing and incarceration are a Band-Aid on issues like poverty and drug use that can trap people in a cycle of poverty.”
Education and impact
Now that the infrastructure for the data hub has been built, and the ICSR Policing team has begun sharing datasets, the next step is for other ICSR teams to start sharing data as well. The cross-disciplinary systemic racism research initiative includes teams working in domains including housing, health care, and social media.
“We want to take advantage of the abundance of data that is available today to answer difficult questions about how racism results from the interactions of multiple systems,” says Munther Dahleh, EECS professor, IDSS founding director, and ICSR co-lead. “Our interest is in how various institutions perpetuate racism, and how technology can exacerbate or combat this.”
To the data hub creators, the main sign of success for the project is seeing the data used in research projects at and beyond MIT. As a resource, though, the hub can support that research for users from a range of experience and backgrounds.
“The data hub is also about education and empowerment,” says Han. “This information can be used in projects designed to teach users how to use big data, how to do data analysis, and even to learn machine learning tools, all specifically to uncover racial disparities in data.”
“Championing the propagation of data skills has been part of the IDSS mission since Day 1,” says Dahleh. “We are excited by the opportunities that making this data available can present in educational contexts, including but not limited to our growing IDSSx suite of online course offerings.”
This emphasis on educational potential only augments the ambitions of ICSR researchers across MIT, who aspire to use data and computing tools to produce actionable insights for policymakers that can lead to real change.
“Systemic racism is an abundantly evidenced societal challenge with far-reaching impacts across domains,” says Christia. “At IDSS, we want to ensure that developing technologies, combined with access to ever-increasing amounts of data, are leveraged to combat racist outcomes rather than continue to enact them.”
October 2, 2024
Data Science and Machine Learning Workshop: UTEC and IDSS/MM SDS
From September 30th to October 2nd, Uruguay Global, an educational program developed by UTEC Uruguay, hosted a 'Data Science and Machine Learning Workshop’ with IDSS, represented by Susana Kevorkova. Umutcan AY,
an IDSS grad student and Teaching Assistant, presented on concepts like exploratory data analysis and generative AI, while leading group projects and final presentations. It was three days of fantastic energy and collaboration!
This is how the Data Science and Machine Learning workshop of the IDSS of the Massachusetts Institute of Technology (MIT) ended the students and graduates of the Certificate in Software Development (CTD).
The course lasted three full days at the LATU Industrialists' Club, with a theoretical and practical approach to the area.
We are especially grateful to the MIT instructor, Umutcan Ay and Susana Kevorkova, representative of the IDSS MicroMasters Program in Statistics and Data Science, and to all of them for participating in a new instance of learning and joint growth.
"This experience personally seemed like a tremendous opportunity to me, I am super satisfied and motivated along with my teammates. I found the presentation we made super enriching (...) Umutcan is very open and has a lot of knowledge about the subject.
We felt the pressure and presence of a course taught by MIT and we understood that the workshop can open the doors to whatever we want." Ignacio Lima, CTD graduate.
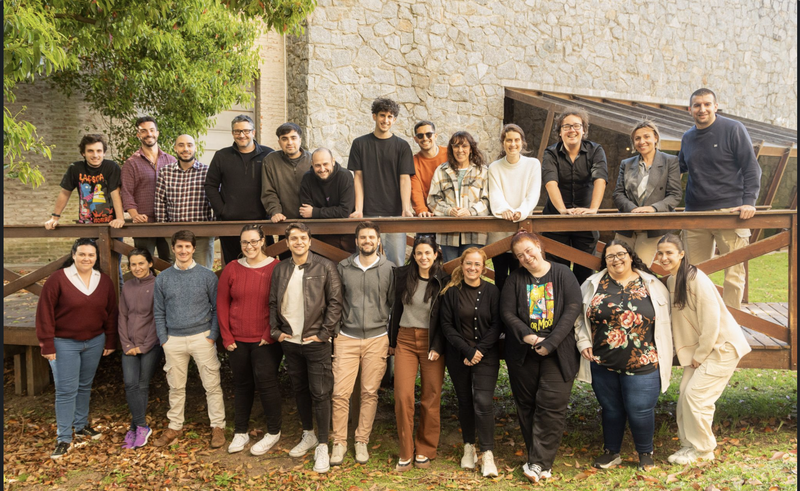
UTEC - Universidad Tecnológica
hashtag#DataScience hashtag#CertifiedTechDeveloper
September 12th, 2024
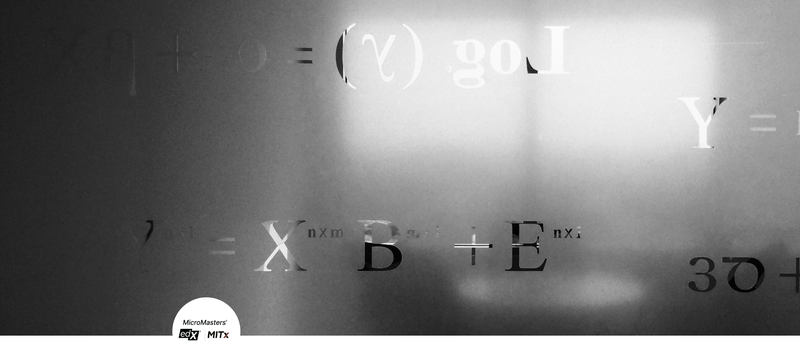
Ask an MIT Professor: Why Data Science Skills Matter in the Age of AI
What value do foundational skills in data science and statistics hold in a world dominated by AI and automation?
The answer might surprise you.
As advanced technologies become more integrated into our lives and work, experts like MIT Professor Devavrat Shah posit that comprehending the mathematical principles behind them is still critically important.
Professor Shah is Faculty Director of the MIT MicroMasters Program in Statistics and Data Science, a multi-track program designed to provide this foundational knowledge.
With advanced tools available, why bother with foundational data science skills?
Professor Shah takes a broad view when considering the value of foundational data science skills in the age of advanced technology. He likens the question of whether we still need to learn basic skills to asking, "If we've already built all the bridges, why would anyone need to study civil engineering? If we've mastered electricity transmission, why do we still train power engineers?"
He draws a parallel with calculators, questioning, “Why teach basic math when we have tools that can do it for us?” The answer, he explains, is clear: foundational skills remain essential.
We still need civil engineers to design and build new bridges and power engineers to innovate and maintain our electrical grids. Children around the world continue to learn math in school because these fundamental concepts are vital, even in a world dominated by advanced tools and technologies.
In the same way, Professor Shah acknowledges that generative AI automates specific tasks, but effectively using this powerful tool requires a deep understanding of where and how to apply it.
“This is why foundational knowledge, such as what is taught at institutions like MIT, remains crucial,” he says. “Just as we need engineers who understand the principles behind bridges and power grids, we need individuals who can grasp the underlying concepts behind AI to truly leverage its potential.”
He adds that a key element of learning is the ability to “tinker.” While automated tools can be efficient in a work environment, there is little room for experimentation. Focusing on foundational knowledge gives learners a “sandbox” where they can tinker and experiment to deepen their understanding.
How does the evolution of technology influence the focus of academic content?
Professor Shah observes that academic content naturally evolves as technology advances.
He uses circuit design as an example. There was a time when electrical engineers and computer scientists spent much of their work learning the mechanics of circuit design. As circuit design became increasingly automated, there was less need for human intervention. However, technology companies need skilled circuit designers to create specialized circuits for modern devices like smartphones. The demand for expertise in specific, high-impact applications is high.
“In that sense, we’ve moved away from teaching some of the nitty-gritty details of circuit design, but we still cover certain aspects,” says Professor Shah. “What we used to teach has been replaced by new content. Over the last 10 to 20 years, much of the teaching in computer science departments has focused on helping people think through how to build AI infrastructure.”
He continues, “As AI infrastructure becomes more consolidated and industry standards emerge, perhaps that’s not what we may end up teaching in the years to come. Maybe we should focus on something else. There will be an evolution, but at the same time, some core elements will remain. For example, we still teach arithmetic, which has endured for thousands of years. Some things are timeless.”
Why is it important to learn foundational concepts in probability and statistics in data science, even with AI tools available?
Professor Shah argues that despite AI's advancements, it has inherent limitations that can lead to significant oversights if users don't grasp the fundamental concepts behind it. He illustrates this idea by highlighting the probabilistic nature of AI and how it contrasts with the deterministic models many of us are accustomed to.
When AI models predict outcomes, they do so based on probabilities rather than certainties. Understanding how to interpret these probabilities is crucial to making informed decisions. Without this nuanced understanding, one might misinterpret AI outputs, leading to flawed decisions or misplaced confidence in the results.
Take weather forecasts as an analogy. When you hear that there’s a 60% chance of rain, it does not simply mean a 60% probability, like flipping a coin. This percentage reflects a complex model that predicts rain in 60% of a given area.
However, the real challenge lies in understanding what probability means in practical terms. It’s not just about the likelihood of rain; it’s also about your confidence in that prediction. If we don't understand these probabilistic concepts, we risk making poor decisions based on AI's predictions and treating them as deterministic when they are not.
The same principles apply to AI in data science. An AI tool might give you a prediction, but without a fundamental understanding of probability and statistics, you might not fully comprehend the confidence level or the potential variance in those predictions. This misstep could lead to significant errors in judgment, especially in fields where precise decision-making is critical.
Therefore, learning foundational data science skills is essential—not only to understand AI's outputs but also to use those outputs effectively and responsibly in real-world applications.
How can the MicroMasters Program in Statistics and Data Science help learners in their professional lives?
Professor Shah highlights that many of us now work in large enterprises that rely on complex departmental and interdepartmental workflows. Decades ago, these workflows were paper-based, with information manually recorded and shared. Over time, digitization transformed these processes, followed by a shift to cloud-based systems.
Now, we're entering a new phase—what he calls “AI infusion” or “AI migration”—where AI is becoming deeply integrated into the processes. The MIT MicroMasters Program in Statistics and Data Science will equip learners with the skills needed to navigate this landscape.
Whether you're a leader driving AI adoption, a manager overseeing its implementation, or a contributor directly involved in using these tools, this program provides the foundational knowledge you need to deliver value.
Prepare Yourself to Lead in an AI-Driven Future
As AI continues to reshape industries, the demand for professionals who can navigate and harness these technologies is only growing. However, understanding the fundamental concepts behind AI tools is just as crucial as knowing how to use them. This knowledge is what enables data scientists to make informed, responsible decisions that drive real impact.
The MIT MicroMasters Program in Statistics and Data Science is designed to prepare you for this challenge. By building a strong foundation in probability, statistics, and data science, you’ll be equipped to lead in a world where technology and data are central to success.
Enroll in the program today and position yourself at the forefront of this evolving landscape.
September 17, 2024
Forecasting a data-driven future
By: Kaitlin Provencher
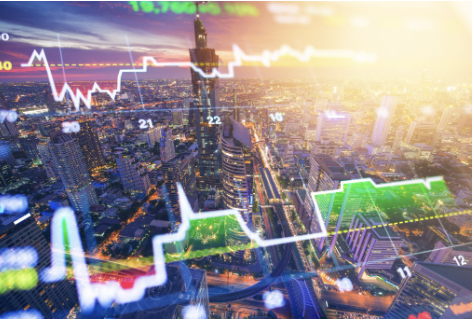
The MicroMasters Program in Statistics and Data Science continues to broaden learners’ skills with the addition of time series analysis and specialized certifications.
“Everything is changing over time,” says Devavrat Shah, director of the MicroMasters Program in Statistics and Data Science (SDS), a statement that not only sets the stage for the program’s newest course, but also the evolution of the MicroMasters program itself.
The online program, created by the MIT Institute for Data, Systems, and Society (IDSS), is now in its sixth year, and will undergo an exciting expansion this fall with the launch of a new course — “Learning Time Series with Interventions” — in addition to the creation of specialized program tracks focused on methods and time series in data science.
With the new course rolling out to learners this month, Assistant Director of Education Karene Chu says the program’s ability to continue to grow is a point of pride for everyone involved.
“We’re really proud that our program continues to evolve. We do not settle with just having a program that works,” Chu says. “Devavrat has envisioned something that will keep expanding to all the different fields relevant to data science.”
She adds: “We are always looking for ways to enrich the program, making it possible for any practicing data scientist to gain a good education, whatever their specific area of interest may be.”
Thinking beyond
As the founding director of MIT’s Statistics and Data Science Center (SDSC) within IDSS, Shah says he was charged with “building, enabling, and empowering academic programs in statistics and data science across the entire campus.” But his personal vision has always been greater.
“I always interpreted that charter a little bit more broadly, feeling that we should figure out how to do this not just within campus, but beyond,” he says.
In 2018, that is what SDSC and IDSS did with the MicroMasters, says Shah: creating an affordable alternative to traditional brick and mortar education while maintaining the standards and high-quality education for which MIT is renowned.
“In an online world we can remove the gates and barriers to entry, creating a meritocratic admission that says if you want to take a class that is the same caliber as what we offer on campus, but you are at a place in life where that is not possible, this will allow that to happen and at a significantly discounted rate,” Shah said. “But know that if you want to get an MIT-level grade A, you will have to work for it. That is the only gate.”
Chu says that each course run sees over 1,500 participants from 100 countries. While the course is asynchronously run, Chu says it is anything but stagnant, thanks to online forums where learners and teaching assistants communicate and work on projects and quizzes throughout the terms.
A time for change
Originally, all program learners took three core methods courses — probability, machine learning, and fundamental statistics — followed by one of two additional options: either a broad data analysis course for those interested in taking a more general path, or data analysis for social scientists for those looking for something more domain specific.
Adding the new time series course is a first step toward putting the program on a new trajectory toward specialized offerings, with four different certification tracks: general, social sciences, methods, or time series and social science combined. Chu says that along with these additions, learners will begin receiving specified certifications which identify the specialization tied to each track they complete.
“Traditionally we’ve spoken about the basic foundations of data science and statistics, but as you go from there to the next layer of complexity, that is when you start thinking about different aspects and dimensions, and how you can control things,” Shah says. “That’s where time series comes in as a natural next step for people to evolve and mature in new directions.”
From probability to predictions
Building from his statement of “everything is changing over time,” Shah says the new course introduces learners to temporal data analysis, learning structured models, predictions, and forecasting.
“With temporal data, the typical first thing to do is figure out if you can forecast things. What’s going to happen tomorrow? Or maybe you want to fill out missing values found in historical data? Or you want to understand what would happen to an outcome if you changed something?”
Some of the first learners to participate in the new course will be members of the Brescia Institute of Technology (BREIT), a cohort of learners that participate in the program as a part of an IDSS partnership with the social impact lab Aporta.
“I expect the course to strengthen my expertise, enabling me to lead more sophisticated projects and contribute to more valuable insights for the company’s growth,” says Josué Mauricio, a 28-year-old learner and machine learning engineer. “It will immediately enhance my ability to handle the large amounts of historical data my company collects, allowing me to create better forecasting models.”
A strong foundation
With the launch of the new course, Shah is already thinking about the next areas of expansion, with potential courses in the disciplines of physics and sports analytics. Expansion aside, Shah says his greatest hope for the program would be to empower the next generation of what he calls the “data science AI workforce.”
Working at the intersection of AI and data science, Shah firmly believes our ability to fully leverage AI’s potential is only possible with a workforce that understands where and how to apply the information it provides. And that understanding can be found in the foundational concepts learners gain from the MicroMasters SDS program.
“AI and data science are different historical disciplines that are accomplishing similar common end goals,” Shah says. “We are trying to enable individuals to use data to obtain better insights, and use those insights to make better decisions. That’s what this MicroMasters program is empowering learners to do.”
He adds: “We need lots of people who are AI savvy for the next 10 years and we’re already in debt to that. If the MicroMasters can play a small role in this bigger issue, I’ll be extremely happy.”
September 12th, 2024
By: MITx MicroMasters® Program Team
Ask an MIT Professor: Why Data Science Skills Matter in the Age of AI
What value do foundational skills in data science and statistics hold in a world dominated by AI and automation?
The answer might surprise you.
As advanced technologies become more integrated into our lives and work, experts like MIT Professor Devavrat Shah posit that comprehending the mathematical principles behind them is still critically important.
Professor Shah is Faculty Director of the MIT MicroMasters Program in Statistics and Data Science, a multi-track program designed to provide this foundational knowledge.
With advanced tools available, why bother with foundational data science skills?
Professor Shah takes a broad view when considering the value of foundational data science skills in the age of advanced technology. He likens the question of whether we still need to learn basic skills to asking, "If we've already built all the bridges, why would anyone need to study civil engineering? If we've mastered electricity transmission, why do we still train power engineers?"
He draws a parallel with calculators, questioning, “Why teach basic math when we have tools that can do it for us?” The answer, he explains, is clear: foundational skills remain essential.
We still need civil engineers to design and build new bridges and power engineers to innovate and maintain our electrical grids. Children around the world continue to learn math in school because these fundamental concepts are vital, even in a world dominated by advanced tools and technologies.
In the same way, Professor Shah acknowledges that generative AI automates specific tasks, but effectively using this powerful tool requires a deep understanding of where and how to apply it.
“This is why foundational knowledge, such as what is taught at institutions like MIT, remains crucial,” he says. “Just as we need engineers who understand the principles behind bridges and power grids, we need individuals who can grasp the underlying concepts behind AI to truly leverage its potential.”
He adds that a key element of learning is the ability to “tinker.” While automated tools can be efficient in a work environment, there is little room for experimentation. Focusing on foundational knowledge gives learners a “sandbox” where they can tinker and experiment to deepen their understanding.
How does the evolution of technology influence the focus of academic content?
Professor Shah observes that academic content naturally evolves as technology advances.
He uses circuit design as an example. There was a time when electrical engineers and computer scientists spent much of their work learning the mechanics of circuit design. As circuit design became increasingly automated, there was less need for human intervention. However, technology companies need skilled circuit designers to create specialized circuits for modern devices like smartphones. The demand for expertise in specific, high-impact applications is high.
“In that sense, we’ve moved away from teaching some of the nitty-gritty details of circuit design, but we still cover certain aspects,” says Professor Shah. “What we used to teach has been replaced by new content. Over the last 10 to 20 years, much of the teaching in computer science departments has focused on helping people think through how to build AI infrastructure.”
He continues, “As AI infrastructure becomes more consolidated and industry standards emerge, perhaps that’s not what we may end up teaching in the years to come. Maybe we should focus on something else. There will be an evolution, but at the same time, some core elements will remain. For example, we still teach arithmetic, which has endured for thousands of years. Some things are timeless.”
Why is it important to learn foundational concepts in probability and statistics in data science, even with AI tools available?
Professor Shah argues that despite AI's advancements, it has inherent limitations that can lead to significant oversights if users don't grasp the fundamental concepts behind it. He illustrates this idea by highlighting the probabilistic nature of AI and how it contrasts with the deterministic models many of us are accustomed to.
When AI models predict outcomes, they do so based on probabilities rather than certainties. Understanding how to interpret these probabilities is crucial to making informed decisions. Without this nuanced understanding, one might misinterpret AI outputs, leading to flawed decisions or misplaced confidence in the results.
Take weather forecasts as an analogy. When you hear that there’s a 60% chance of rain, it does not simply mean a 60% probability, like flipping a coin. This percentage reflects a complex model that predicts rain in 60% of a given area.
However, the real challenge lies in understanding what probability means in practical terms. It’s not just about the likelihood of rain; it’s also about your confidence in that prediction. If we don't understand these probabilistic concepts, we risk making poor decisions based on AI's predictions and treating them as deterministic when they are not.
The same principles apply to AI in data science. An AI tool might give you a prediction, but without a fundamental understanding of probability and statistics, you might not fully comprehend the confidence level or the potential variance in those predictions. This misstep could lead to significant errors in judgment, especially in fields where precise decision-making is critical.
Therefore, learning foundational data science skills is essential—not only to understand AI's outputs but also to use those outputs effectively and responsibly in real-world applications.
How can the MicroMasters Program in Statistics and Data Science help learners in their professional lives?
Professor Shah highlights that many of us now work in large enterprises that rely on complex departmental and interdepartmental workflows. Decades ago, these workflows were paper-based, with information manually recorded and shared. Over time, digitization transformed these processes, followed by a shift to cloud-based systems.
Now, we're entering a new phase—what he calls “AI infusion” or “AI migration”—where AI is becoming deeply integrated into the processes. The MIT MicroMasters Program in Statistics and Data Science will equip learners with the skills needed to navigate this landscape.
Whether you're a leader driving AI adoption, a manager overseeing its implementation, or a contributor directly involved in using these tools, this program provides the foundational knowledge you need to deliver value.
Prepare Yourself to Lead in an AI-Driven Future
As AI continues to reshape industries, the demand for professionals who can navigate and harness these technologies is only growing. However, understanding the fundamental concepts behind AI tools is just as crucial as knowing how to use them. This knowledge is what enables data scientists to make informed, responsible decisions that drive real impact.
The MIT MicroMasters Program in Statistics and Data Science is designed to prepare you for this challenge. By building a strong foundation in probability, statistics, and data science, you’ll be equipped to lead in a world where technology and data are central to success.
Enroll in the program today and position yourself at the forefront of this evolving landscape.
August 20, 2024
MITx: Learning Time Series with Interventions
MM SDS has launched a new course: Learning Time Series with Interventions offers an in-depth introduction to time series analysis, from learning structured models to predictions and reinforcement learning, with hands-on projects.
What you'll learn:
- Analyze time series through the perspective of Linear Time-invariant (LTI) systems and use methods and tools such as spectral analysis.
- Model time series using autoregressive moving average (ARMA) and integrated processes.
- Perform prediction, imputation on general time series data using matrix completion methods.
- Use various dynamical programming and reinforcement learning algorithms to optimize control and interventions for time series.
August 20, 2024
MM SDS TRACKS - UPDATED
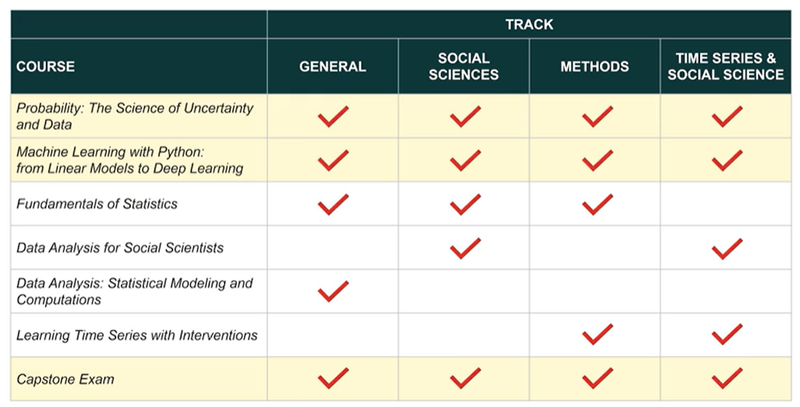
General Track
This track will prepare you to become an informed and effective practitioner of data science who adds value to your organization across industries.
Explore the General track here
Methods Track
This track will prepare you with in-depth knowledge of data science and time series analysis and will enable you to conduct rigorous analysis, inform decision-making processes, and contribute to evidence-based practices across industries.
Explore the Methods track here
Social Sciences Track
This track will prepare you to extract meaningful insights from social, cultural, economic, and policy-related data and equip you to tackle complex real-world problems and contribute to cutting-edge advancements in AI and data-driven solutions within all social sciences.
Explore the Social Sciences track here
Time Series and Social Sciences Track (New)
This track will equip you to analyze the impact of interventions on time series data, preparing you for roles in economics, public policy, and social sciences where understanding temporal dynamics is crucial for informed decision-making and policy formulation.
Explore the Time Series and Social Sciences track here
All tracks are taught by MIT faculty and administered by IDSS at a similar pace and level of rigor as an on-campus course at MIT. The program is designed for learners who want to acquire sophisticated and rigorous training in data science without leaving their day job but without compromising quality.
There is no application process, but college-level calculus and comfort with mathematical reasoning and Python programming are highly recommended if you want to excel.
MITx MicroMasters credential leads learner to accelerated graduate program in data science.
Publication Date: December 15, 2023
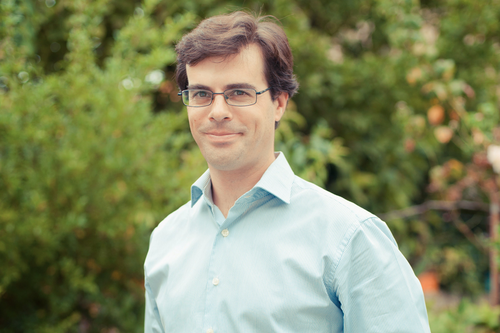
Earning his MicroMasters credential not only gave da Silva Branco Magalhães a strong foundation to tackle more complex transportation problems, but it also opened the door to pursuing an accelerated graduate degree via a Northwestern University online program.
Learners who earn their MicroMasters credentials gain the opportunity to apply to and continue their studies at a pathway school. The MicroMasters in Statistics and Data Science credential can be applied as credit for a master’s program at more than 30 universities,
as well as MIT’s PhD Program in Social and Engineering Systems. Da Silva Branco Magalhães, originally from Portugal and now based in Australia, seized this opportunity and enrolled in Northwestern University’s Master’s in Data Science for MIT MicroMasters Credential Holders.
The article continues here.
DEMOCRATIZING EDUCATION: BRINGING MIT EXCELLENCE TO THE MASSES
By Kaitlin Provencher | Institute for Data, Systems, and Society
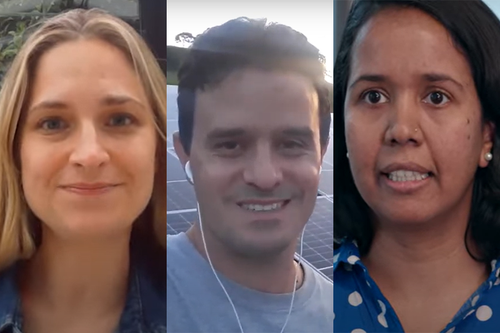
How do you quantify the value of education or measure success? For the team behind the Institute for Data, Systems, and Society’s (IDSS) MicroMasters® Program in Statistics and Data Science (SDS), providing over 1,000 individuals from around the globe with access to MIT-level programming feels like a pretty good place to start.
Thanks to the MIT-conceived MicroMasters-style format, SDS Faculty Director Devavrat Shah and his colleagues have eliminated the physical restrictions created by a traditional brick-and-mortar education, allowing 1,178 learners and counting from 89 countries access to an MIT education.
The article continues here.
MICROMASTERS LEARNERS FROM UTEC URUGUAY VISIT MIT
Publication Date: January 30, 2023
The partnership between IDSS and UTEC Uruguay supports graduate students enrolled in the UTEC Program in Data Science, including tailored support for the IDSS-created MicroMasters Program in Statistics and Data Science. In October of 2022, learners from UTEC visited the MIT campus to celebrate their completion of the program.
The UTEC Program in Data Science is a blended learning program that builds upon the MicroMasters Program in Data Science and Statistics. After completing four courses and a capstone exam, successful students transition to the subsequent residential master’s program at UTEC. UTEC is an MITx global pathway school, one of many worldwide offering credit toward the completion of a graduate degree to credential holders of the MicroMasters program.
The article continueshere.
Research, education, and connection in the face of war
"I wouldn’t let the aggressor in the war squash my dreams,” says Ukrainian mathematician and MITx MicroMasters learner Tetiana Herasymova. That’s why Herasymova sought out the MITxMicroMasters Program in Statistics and Data Science offered by the MIT Institute for Data, Systems, and Society (IDSS).”
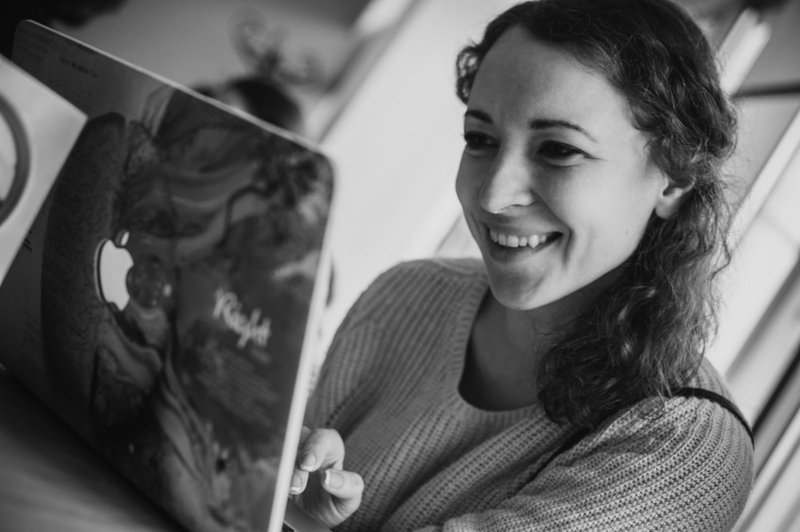
Tetiana Herasymova registered for her MITx program’s final exams just days prior to moving into a bomb shelter. Despite war all around her, Herasymova remained determined to complete her goal — she passed all of her exams in May, the final step to earning her MITx MicroMasters certificate in statistics and data science.
The article continueshere.
Podcast: Data Nation
Publication Date: September 29, 2022
IDSS takes a holistic and data-driven approach to analyzing complex, high-impact systems in society. In discussing the challenges our society faces – systemic racism, data privacy, misinformation, among others – we rely on expertise and knowledge from a variety of disciplines.
In Data Nation, the podcast produced in collaboration with Voxtopica, our faculty engages in conversations with industry experts on how to find solutions for these problems. Each episode unpacks a different example of how data can be used to lead, mislead, manipulate, and inform the public’s viewpoints and decisions.
We face many overwhelming challenges in America today: systemic racism, data privacy, and political misinformation. These are big problems, and there are a lot of opinions and ideas on how to fix them. Scholars and industry experts often disagree on how to find solutions. So, how can we find the right way to move forward? We let the data speak for itself. Join hosts Liberty Vittert and Scott Tranter as they gather data and get the facts about today’s most pressing problems to find out: are solutions even possible? They’ll investigate with MIT professors dedicated to researching these issues, and talk with the people on the ground encountering these problems every day so that we can find the best solutions that triumph over these challenges and solve America’s biggest problems.
Find new episodes here every Thursday, or subscribe to the podcast feed on Apple, Spotify, or any other podcast platform.
Online MM Completion Celebration Update
Publication Date: July 12, 2022
2022 MM SDS Presenters - Completion Ceremony
MIT Open Learning and the MicroMasters Statistics and Data Science Program (SDS) are proud to share with you the link for our third MM Completion Celebration, which occurred virtually on June 23, 2022.
Featured are three recent MM SDS credential holders who have shared their experiences in the program.
Supporting Women in Data Science
Publication date:January 26, 2022
We are excited to engage in a special campaign that aims to encourage learners who identify as women to participate in the MicroMasters Program in Statistics and Data Science.
This campaign is set to launch with Probability- The Science of Uncertainty and Data, a natural entry point into the MicroMasters Program in SDS, starting on January 31, 2022. The MM SDS program will offer limited scholarships in the form of 25% discount codes to select participants who identify as women. The discount code may be used towards verification within the Probability course.
Online MM Completion Celebration Update
Publication date: July 22, 2021
MIT Open Learning and the MicroMasters Statistics and Data Science Program (SDS) are proud to share the link for our second MM Completion Celebration, which occurred virtually on July 9, 2021.
Over 700 learners and their families watched the show live.
The event recording includes brief remarks from IDSS leaders and MIT faculty, including MicroMasters Program in Statistics and Data Science Director Devavrat Shah. Also featured are three recent MM SDS credential holders who have shared their experiences in the program.
We are very proud of all learners and look forward to celebrating next years group!
Note — The SDS portion of the video begins at approximately 33:00 through 44:00.
MITx MicroMasters Program in Statistics and Data Science announces new Data Analysis elective
Publication date: February, 2021
New course focuses on hands-on applications of data analysis across disciplines
A new elective course in the MITx MicroMasters Program in Statistics and Data Science (SDS) offers an increased focus on applying data science to complex, real-world problems. Data Analysis: Statistical Modeling and Computation in Applications launched February 15, 2021, and is open for enrollment now.
Read more on the new elective course Data Analysis: Statistical Modeling and Computation in Applications in the MIT Open Learning article here.
Note: If you have already purchased the MITx MicroMasters Program Bundle with the elective course 14.310Fx, you will need to contact edX customer service team if you wish to take the new 6.419x elective course instead.
Celebration Times: IDSS Educational Partnership with Aporta Celebrates the First Cohort of Graduates in Peru
Publication date: February, 2021
On February 6, 2021 Aporta and MITx MicroMasters Program in Statistics and Data Science (MM SDS) came together (virtually) to celebrate the learners of Cohort 1 as they completed 18 months of intensive learning of completing the MM SDS credential.
In the spring of 2019, Peruvian social impact group Aporta launched the Advanced Program in Data Science and Global Skills. Through an education partnership with IDSS, these learners take courses in probability, statistics, machine learning, and data analysis offered through the MIT MicroMasters in Statistics and Data Science.
Read all about the partnership with Aporta and MM SDS in the IDSS article A TRAIL-BLAZING COHORT.
MAKING DATA-INFORMED COVID-19 TESTING PLANS
Publication date: February, 2021
A new web Covid-19 Testing Impact Calculator at WhenToTest.org has been developed by MIT researchers with the Institute for Data, Systems, and Society (IDSS), in collaboration with the Consortia for Improving Medicine with Innovation & Technology (CIMIT), to help organizations around the world answer these questions.
November, 2020
Investment and Advancement is Key: MIT Professor Devavrat Shah on the Recommendation Engine Industry of the Future
An exciting new article, Investment and Advancement is Key: MIT Professor Devavrat Shah on the Recommendation Engine Industry of the Future is out and you can read the full article on MIT xPRO here.
Below is an excerpt form the article:
The market for recommendation engines is predicted to grow by USD $3.57B in the next four years, according to a recent report from Technavio. What’s the story behind these growth numbers? We talked with MIT Professor of Electrical Engineering & Computer Science and co-founder of machine learning startup Celect, Devavrat Shah, about the findings from this recent report and to discuss his predictions for recommendation systems technology.
We’re all familiar with Amazon recommendations or the carousel of curated shows and movies that appear on your Netflix profile. You have likely interacted with a recommendation engine if you researched a school, searched for a new dentist, or visited a dating site. And there are a multitude of opportunities beyond consumer applications, for everything from government policy and supply chain to market dynamics and competitive landscape.
"What gets more meaningful results is offering options, like an optician with lenses. Which is better, this one or that one? And it’s not just important what you buy, but what you don’t buy.” -- Professor Devavrat Shah
September, 2020
Regina Barzilay - Course Faculty for Machine Learning course in MicroMasters Program in Statistics and Data Science (MM SDS) -- wins $1M Association for the Advancement of Artificial Intelligence Squirrel AI award.
MIT professor announced as award’s first recipient for work in cancer diagnosis and drug synthesis.
Read the full story at MIT news!
We are excited to announce and share that MM SDS professor Regina Barzilay was awarded $1M by the Association for the Advancement of Artificial Intelligence Squirrel AI award. Professor Regina Barzilay is the first recipient of the Squirrel AI Award for Artificial Intelligence for the Benefit of Humanity.
For more than 100 years Nobel Prizes have been given out annually to recognize breakthrough achievements in chemistry, literature, medicine, peace, and physics. As these disciplines undoubtedly continue to impact society, newer fields like artificial intelligence (AI) and robotics have also begun to profoundly reshape the world.
In recognition of this, the world’s largest AI society — the Association for the Advancement of Artificial Intelligence (AAAI) — announced today the winner of their new Squirrel AI Award for Artificial Intelligence for the Benefit of Humanity, a $1 million award given to honor individuals whose work in the field has had a transformative impact on society.
The recipient, Regina Barzilay, the Delta Electronics Professor of Electrical Engineering and Computer Science at MIT and a member of MIT’s Computer Science and Artificial Intelligence Laboratory (CSAIL), is being recognized for her work developing machine learning models to develop antibiotics and other drugs, and to detect and diagnose breast cancer at early stages.
In February, AAAI will officially present Barzilay with the award, which comes with an associated prize of $1 million provided by the online education company Squirrel AI.
September, 2020
New Data Analysis Elective - 6.419x Data Analysis: Statistical Modeling and Computation in Applications
The MicroMasters Program in Statistics and Data Science is excited to announce that starting Spring 2021, a new data analysis course---Data Analysis: Statistical Modeling and Computation in Applications, will become an additional offering as part of the MicroMaster Program in Statistics and Data Science.
This new course is a hands-on introduction to the interplay between statistics and computation for the analysis of real data. Learners will apply their fundamental knowledge on randomized control trials, hypothesis testing, linear regression, principal component analysis, and learn and implement common models and methods to analyze specific types of data in four different domain areas:
- Epigenetic Codes and Data Visualization
- Criminal Networks and Network Analysis
- Prices, Economics and Time Series
- Environmental Data and Spatial Statistics
Each module will have homework that will guide learners to analyze a real data set from the domain of focus. They will use python, R or other software of their choice to perform full analysis and improve their data visualization and communication skills through written reports in homeworks.
Elective Structure
6.419x Data Analysis: Statistical Modeling and Computation in Applications will be an elective course in the MITx MicroMasters Program in Statistics and Data Science, juxtaposed against 14.310/14.310Fx Data Analysis for Social Scientists/Data Analysis in Social Sciences--Assessing your Knowledge. See here for more information on the course.
What this means is that to complete the SDS MicroMasters Program, learners will need to take the 3 core courses, and 1 elective; and for the elective, learners can choose either 14.310x Data Analysis in Social Science—Assessing Your Knowledge /14.310Fx Data Analysis in Social Science—Assessing Your Knowledge, or the new elective course 6.419x Data Analysis: Statistical Modeling and Computation in Applications. Once learners have passed their 4 courses (3 core +1 elective), they will then take the virtually-proctored Capstone exam to earn the MicroMasters in Statistics and Data Science credential.
Dates
The new data analysis elective will open to learners with its first run February 2021 - May 2021, with registration and exact course dates sometime in Fall 2020. Please check the MM SDS schedule and Dates FAQ page for future updates.
July 28, 2020
Online MM Completion Celebration Update
MIT Open Learning and the MicroMasters Statistics and Data Science Program (SDS) are proud to share with you the link below for our first MM Completion Celebration, which occurred virtually on June 18, 2020. After six months planning, we launched our first joint MM (PoM, DEDP, SDS) completion celebration. Close to 500 learners and their families watched the show live.
The event recording includes brief remarks from IDSS leaders and MIT faculty, including IDSS Director Munther Dahleh and MicroMasters Program in Statistics and Data Science Director Devavrat Shah. Also featured are a selection of MM SDS credential holders who have shared their experiences in the program.
Read all about the MM Completion Celebration here in this MIT News article, "Learners today, leaders tomorrow."
You can also view on demand a video recording of the inaugural celebration here.
Note — The SDS portion of the video begins at 23:54 through 38:46.
We are very proud of all learners and look forward to celebrating next years group!